1.3.4. 高级操作¶
1.3.4.1. 多项式¶
NumPy 还包含不同基底的多项式
例如,
>>> p = np.poly1d([3, 2, -1])
>>> p(0)
np.int64(-1)
>>> p.roots
array([-1. , 0.33333333])
>>> p.order
2
>>> x = np.linspace(0, 1, 20)
>>> rng = np.random.default_rng()
>>> y = np.cos(x) + 0.3*rng.random(20)
>>> p = np.poly1d(np.polyfit(x, y, 3))
>>> t = np.linspace(0, 1, 200) # use a larger number of points for smoother plotting
>>> plt.plot(x, y, 'o', t, p(t), '-')
[<matplotlib.lines.Line2D object at ...>, <matplotlib.lines.Line2D object at ...>]
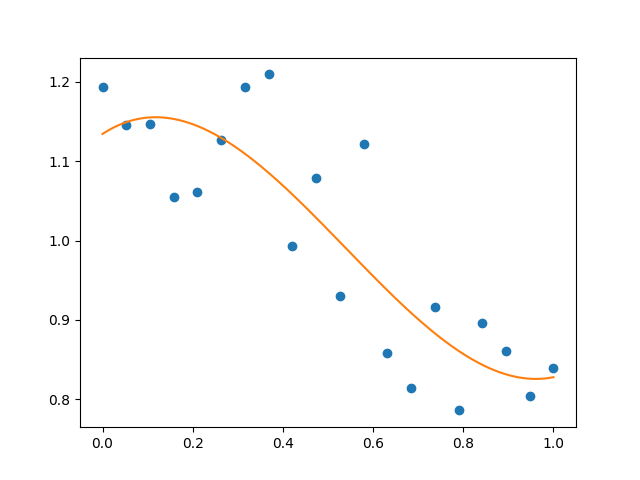
更多信息请参见 https://numpy.com.cn/doc/stable/reference/routines.polynomials.poly1d.html。
更多多项式(更多基底)¶
NumPy 还提供了一个更复杂的多项式接口,支持例如切比雪夫基底。
:
>>> p = np.polynomial.Polynomial([-1, 2, 3]) # coefs in different order!
>>> p(0)
np.float64(-1.0)
>>> p.roots()
array([-1. , 0.33333333])
>>> p.degree() # In general polynomials do not always expose 'order'
2
使用切比雪夫基底的多项式的示例,适用于区间 [-1, 1]
中的多项式
>>> x = np.linspace(-1, 1, 2000)
>>> rng = np.random.default_rng()
>>> y = np.cos(x) + 0.3*rng.random(2000)
>>> p = np.polynomial.Chebyshev.fit(x, y, 90)
>>> plt.plot(x, y, 'r.')
[<matplotlib.lines.Line2D object at ...>]
>>> plt.plot(x, p(x), 'k-', lw=3)
[<matplotlib.lines.Line2D object at ...>]
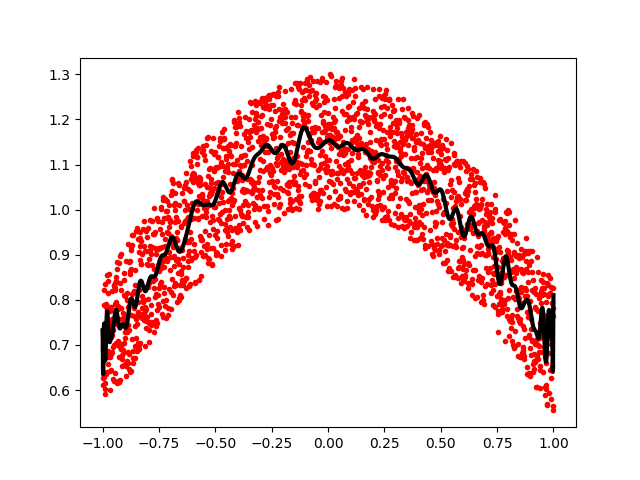
切比雪夫多项式在插值方面具有一些优势。
1.3.4.2. 加载数据文件¶
文本文件¶
示例: populations.txt
# year hare lynx carrot 1900 30e3 4e3 48300 1901 47.2e3 6.1e3 48200 1902 70.2e3 9.8e3 41500 1903 77.4e3 35.2e3 38200
>>> data = np.loadtxt('data/populations.txt')
>>> data
array([[ 1900., 30000., 4000., 48300.],
[ 1901., 47200., 6100., 48200.],
[ 1902., 70200., 9800., 41500.],
...
>>> np.savetxt('pop2.txt', data)
>>> data2 = np.loadtxt('pop2.txt')
注意
如果您的文本文件比较复杂,您可以尝试以下方法:
np.genfromtxt
使用 Python 的 I/O 函数和例如正则表达式进行解析(Python 非常适合此任务)
图像¶
使用 Matplotlib
>>> img = plt.imread('data/elephant.png')
>>> img.shape, img.dtype
((200, 300, 3), dtype('float32'))
>>> plt.imshow(img)
<matplotlib.image.AxesImage object at ...>
>>> plt.savefig('plot.png')
>>> plt.imsave('red_elephant.png', img[:,:,0], cmap=plt.cm.gray)
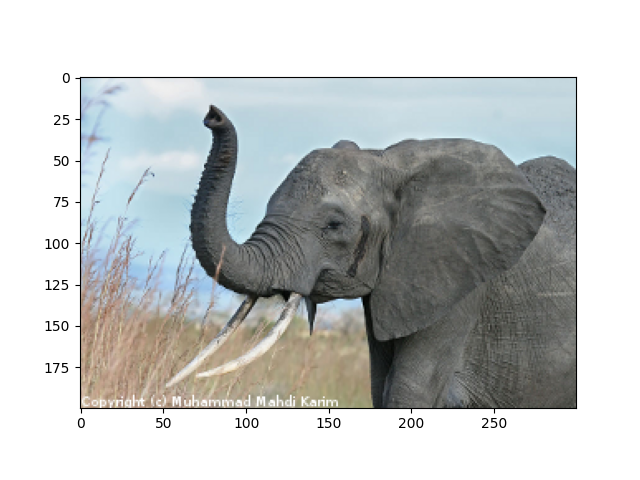
这仅保存了一个通道(RGB 中的一个)。
>>> plt.imshow(plt.imread('red_elephant.png'))
<matplotlib.image.AxesImage object at ...>
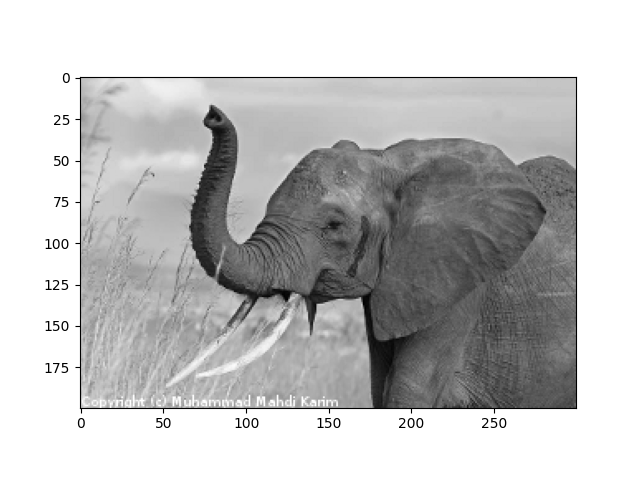
其他库
>>> import imageio.v3 as iio
>>> iio.imwrite('tiny_elephant.png', (img[::6,::6] * 255).astype(np.uint8))
>>> plt.imshow(plt.imread('tiny_elephant.png'), interpolation='nearest')
<matplotlib.image.AxesImage object at ...>
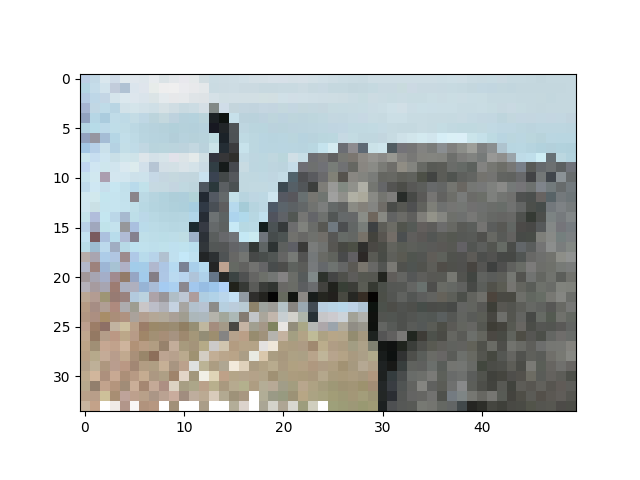
NumPy 自身格式¶
NumPy 有自己的二进制格式,不具备可移植性,但 I/O 效率很高。
>>> data = np.ones((3, 3))
>>> np.save('pop.npy', data)
>>> data3 = np.load('pop.npy')
常用(和鲜为人知)的文件格式¶
NetCDF:
scipy.io.netcdf_file
, netcdf4-python,…Matlab:
scipy.io.loadmat
,scipy.io.savemat
MatrixMarket:
scipy.io.mmread
,scipy.io.mmwrite
IDL:
scipy.io.readsav
… 如果有人使用它,可能也存在一个 Python 库。