注意
转到末尾 下载完整的示例代码。
3.4.8.13. 数字数据集的简单可视化和分类¶
绘制数字数据集的前几个样本和使用 PCA 构建的二维表示,然后进行简单的分类
from sklearn.datasets import load_digits
digits = load_digits()
绘制数据:数字图像¶
每个数据都是一个 8x8 的图像
import matplotlib.pyplot as plt
fig = plt.figure(figsize=(6, 6)) # figure size in inches
fig.subplots_adjust(left=0, right=1, bottom=0, top=1, hspace=0.05, wspace=0.05)
for i in range(64):
ax = fig.add_subplot(8, 8, i + 1, xticks=[], yticks=[])
ax.imshow(digits.images[i], cmap="binary", interpolation="nearest")
# label the image with the target value
ax.text(0, 7, str(digits.target[i]))
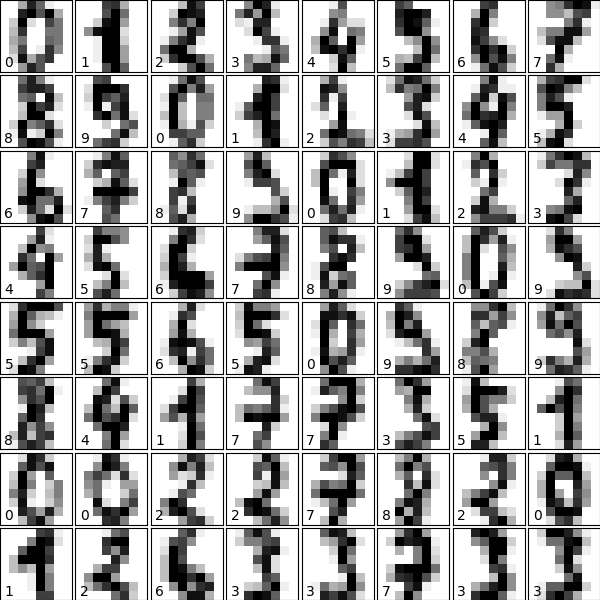
绘制前两个主成分轴上的投影¶
plt.figure()
from sklearn.decomposition import PCA
pca = PCA(n_components=2)
proj = pca.fit_transform(digits.data)
plt.scatter(proj[:, 0], proj[:, 1], c=digits.target, cmap="Paired")
plt.colorbar()
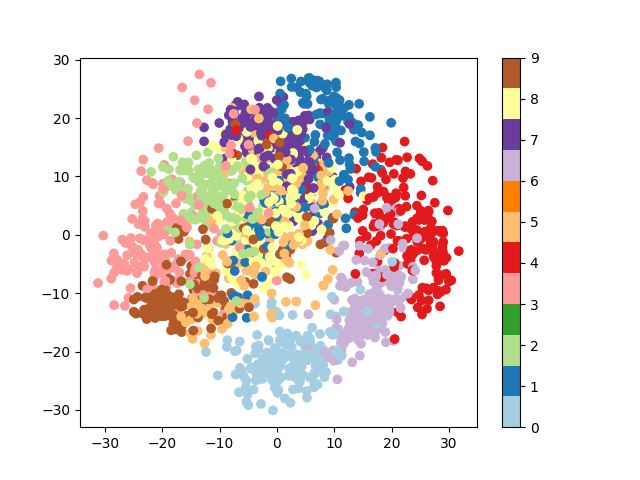
<matplotlib.colorbar.Colorbar object at 0x7f78e6f3eff0>
使用高斯朴素贝叶斯进行分类¶
from sklearn.naive_bayes import GaussianNB
from sklearn.model_selection import train_test_split
# split the data into training and validation sets
X_train, X_test, y_train, y_test = train_test_split(digits.data, digits.target)
# train the model
clf = GaussianNB()
clf.fit(X_train, y_train)
# use the model to predict the labels of the test data
predicted = clf.predict(X_test)
expected = y_test
# Plot the prediction
fig = plt.figure(figsize=(6, 6)) # figure size in inches
fig.subplots_adjust(left=0, right=1, bottom=0, top=1, hspace=0.05, wspace=0.05)
# plot the digits: each image is 8x8 pixels
for i in range(64):
ax = fig.add_subplot(8, 8, i + 1, xticks=[], yticks=[])
ax.imshow(X_test.reshape(-1, 8, 8)[i], cmap="binary", interpolation="nearest")
# label the image with the target value
if predicted[i] == expected[i]:
ax.text(0, 7, str(predicted[i]), color="green")
else:
ax.text(0, 7, str(predicted[i]), color="red")
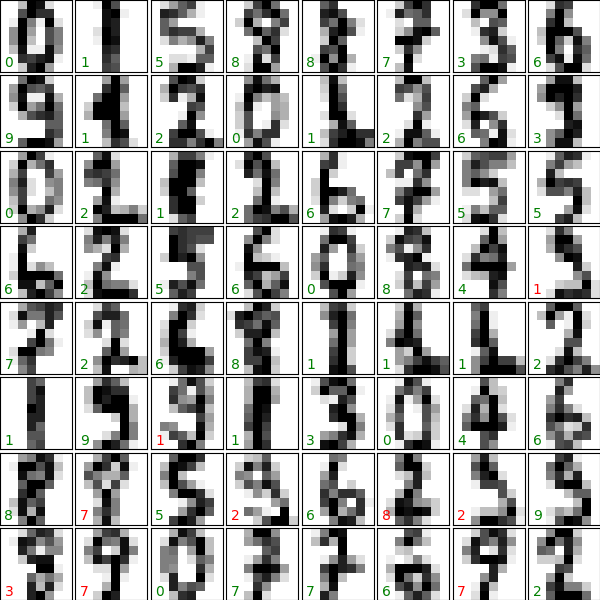
量化性能¶
首先打印正确匹配的数量
395
数据点的总数
print(len(matches))
450
现在,打印正确预测的比率
np.float64(0.8777777777777778)
打印分类报告
from sklearn import metrics
print(metrics.classification_report(expected, predicted))
precision recall f1-score support
0 0.97 0.95 0.96 37
1 0.83 0.85 0.84 41
2 0.89 0.84 0.86 49
3 0.93 0.83 0.88 47
4 0.93 0.90 0.92 42
5 0.89 0.95 0.92 42
6 0.98 0.97 0.97 60
7 0.81 0.98 0.88 47
8 0.65 0.87 0.75 39
9 0.97 0.63 0.76 46
accuracy 0.88 450
macro avg 0.89 0.88 0.87 450
weighted avg 0.89 0.88 0.88 450
打印混淆矩阵
print(metrics.confusion_matrix(expected, predicted))
plt.show()
[[35 0 0 0 1 0 0 1 0 0]
[ 0 35 0 0 0 0 1 1 4 0]
[ 0 1 41 0 0 0 0 0 7 0]
[ 0 0 2 39 0 1 0 2 2 1]
[ 0 1 0 0 38 0 0 2 1 0]
[ 0 0 0 0 1 40 0 1 0 0]
[ 0 0 1 0 1 0 58 0 0 0]
[ 0 0 0 0 0 1 0 46 0 0]
[ 0 2 0 1 0 1 0 1 34 0]
[ 1 3 2 2 0 2 0 3 4 29]]
脚本总运行时间:(0 分钟 1.696 秒)